
Table of Contents
ToggleIntroduction
In the dynamic landscape of Recruitment Process Outsourcing (RPO), the integration of data analytics marks a transformative era. This integration heralds a shift towards predictive hiring, revolutionizing how companies source talent and plan their workforce. The blog post ahead delves into the pivotal role of data analytics in RPO, highlighting its impact on predictive hiring strategies and the future of talent sourcing. We explore how leveraging data-driven insights not only refines recruitment processes but also enhances the efficiency, accuracy, and overall success of hiring initiatives. By harnessing the power of data, RPO is moving towards more informed, strategic, and customized talent acquisition approaches. This change is not just a trend but a necessary evolution in the face of a rapidly changing employment landscape, where the ability to predict and adapt becomes a key differentiator in the competitive world of talent sourcing.
1. Introduction to Data Analytics in RPO
- Data-Driven Decision Making: Utilizing data analytics for informed decision-making in recruitment processes.
- Enhancing Recruitment Efficiency: Streamlining hiring processes through predictive analytics.
- Customized Candidate Experience: Tailoring recruitment strategies to individual candidate profiles.
- Strategic Talent Sourcing: Leveraging data for targeted talent acquisition and workforce planning.
- Competitive Advantage: Gaining an edge in talent sourcing through analytical insights.
For a comprehensive understanding of RPO benefits, consider reading Benefits of Having Recruitment Process Outsourcing on Your Side.
We explore how leveraging data-driven insights not only refines recruitment processes but also enhances the efficiency, accuracy, and overall success of hiring initiatives. By harnessing the power of data, RPO is moving towards more informed, strategic, and customized talent acquisition approaches.
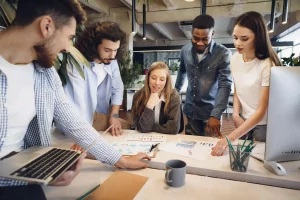
2. The Role of Predictive Analytics in Hiring
- Forecasting Hiring Needs: Predicting future talent requirements based on company data.
- Identifying Candidate Success Factors: Analyzing characteristics of successful hires to improve future recruitment.
- Reducing Hiring Risks: Mitigating the risks of bad hires through predictive insights.
- Streamlining Talent Pipeline: Efficiently managing the talent pipeline with predictive models.
- Enhancing Diversity and Inclusion: Using data to promote diverse hiring practices.
Explore the significance of diversity in recruitment with Can RPO Services Enhance Workplace Diversity?.
3. Integrating AI and Machine Learning in RPO
- Automated Candidate Screening: Implementing AI for efficient resume screening and candidate evaluation.
- Enhanced Candidate Matching: Utilizing machine learning algorithms for precise candidate-job matching.
- Predictive Candidate Engagement: Forecasting candidate behaviors and preferences for better engagement strategies.
- Continuous Learning and Improvement: AI systems learning from ongoing recruitment processes for enhanced outcomes.
- Data Security and Compliance: Ensuring data protection and regulatory compliance in AI-driven recruitment.
For insights into the role of technology in RPO, see Understanding Using RPO Technology: A Digital Revolution in the Making.
4. The Impact of Big Data on Talent Acquisition
- Volume and Variety of Data: Harnessing vast amounts of data from diverse sources for deeper insights.
- Real-Time Analytics: Using real-time data for dynamic talent sourcing strategies.
- Customized Recruitment Campaigns: Designing data-driven, targeted recruitment marketing campaigns.
- Predictive Workforce Planning: Utilizing big data for strategic workforce development and planning.
- Enhanced Candidate Profiling: Creating comprehensive candidate profiles for better fit analysis.
Discover more about talent sourcing strategies at Talent Solutions: A Paradigm Shift in the Industry.
5. Advantages of Predictive Hiring in RPO
- Data Input: Quality and quantity of data fed into the system determines AI performance.
- Continuous Learning: AI systems require regular updates and learning for improved functionality.
- Ethical Considerations: Training AI with ethical guidelines to avoid biases.
- User Experience: Focus on creating user-friendly interfaces for both candidates and recruiters.
- Feedback Loop: Incorporating feedback to refine AI and chatbot functionalities.
6. Challenges and Solutions in Implementing Predictive Analytics
- Data Quality and Integration: Ensuring high-quality, integrated data for accurate predictions.
- Balancing Human and Machine Intelligence: Combining human expertise with AI for optimal results.
- Privacy and Ethical Considerations: Addressing privacy concerns and ethical implications of data usage.
- Upgrading Technical Infrastructure: Investing in the necessary technology and tools for effective data analysis.
- Continuous Learning and Adaptation: Regularly updating and refining predictive models for relevance.
Learn about overcoming recruitment challenges at Recruitment Challenges: Why Has Recruitment Become Harder?.
7. Case Studies: Success Stories in Predictive Hiring
- Improved Candidate Fit: Examples of companies enhancing candidate-job fit through predictive analytics.
- Efficient High-Volume Hiring: Success in managing large-scale recruitment efficiently with data analytics.
- Reduced Employee Turnover: How predictive hiring has led to lower turnover rates.
- Enhanced Employer Branding: Boosting employer brand through data-driven recruitment strategies.
- Diversity and Inclusion Successes: Case studies showcasing improved diversity in hiring through analytics.
For insights into RPO success, visit Top 5 Positive Side-Effects You Reap After Using an RPO Service.
8. The Future of Talent Sourcing with Data Analytics
- Continuous Evolution of AI and ML: The ongoing development of AI and ML in enhancing recruitment processes.
- Predictive Analytics as a Standard Practice: The increasing adoption of predictive analytics in RPO.
- Enhanced Global Talent Pool Access: Leveraging data for global talent sourcing and acquisition.
- Customization of Candidate Experiences: Providing personalized candidate experiences based on data insights.
- Integration with Other HR Functions: The role of data analytics in broader HR strategy and operations.
Explore future RPO trends at RPO Trends to Keep an Eye On in 2022.
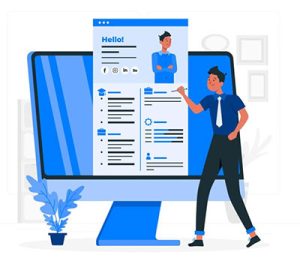
Predictive analytics enhances recruitment by forecasting hiring needs, reducing hiring risks, and improving candidate-job matching, leading to better quality hires and reduced time-to-hire.
Conclusion: Embracing Predictive Hiring in RPO
In conclusion, the integration of predictive hiring into Recruitment Process Outsourcing (RPO) signifies a major leap forward in talent acquisition. This approach, driven by data analytics, offers a strategic advantage by aligning recruitment with business goals and market dynamics. It emphasizes the importance of continuous learning and adaptation to stay ahead in a rapidly evolving job market. The collaboration between data experts and talent professionals is crucial, ensuring that recruitment strategies are not only data-driven but also human-centric. Ethical considerations and responsible data usage remain paramount to maintain trust and compliance. Investing in predictive analytics is not merely a temporary measure but a long-term commitment to revolutionizing recruitment processes. It’s an investment in the future of talent sourcing, where data intelligence and human expertise combine to create a more efficient, effective, and ethical recruitment landscape.